Ep 75 - Critical Appraisal Nugget 1: Randomisation
The St.Emlyn’s Podcast - Un podcast de St Emlyn’s Blog and Podcast - Les mercredis
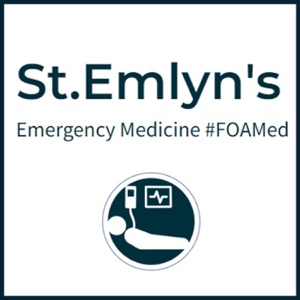
Catégories:
Understanding Randomization in Clinical Trials: A Guide for Critical Appraisal Welcome to the St. Emlyn's blog, your go-to resource for insights into emergency medicine and critical care. Today, we're diving into a crucial aspect of clinical research: randomization. Whether you're preparing for exams like the FR-KEM or just want to deepen your understanding of clinical trials, understanding randomization is key to critical appraisal. This blog post will walk you through the essentials, common pitfalls, and best practices for ensuring robust study design. What is Randomization? Randomization is a foundational process in clinical trials, particularly those evaluating interventions. It refers to the random allocation of participants into different treatment groups. This process aims to eliminate selection bias and ensure that differences in outcomes can be attributed to the intervention itself rather than other factors. Why is Randomization Important?Randomization is crucial because it helps establish causality. Without it, studies might only reveal associations rather than true cause-and-effect relationships. For example, if we observe patients receiving different treatments in a non-randomized manner, systematic differences between the groups—such as varying standards of care—could confound the results. Randomization seeks to balance these factors, allowing for a clearer interpretation of the intervention's effectiveness. Key Components of Randomization Random Allocation: This is the process of assigning participants to treatment groups purely by chance. It can be done using random number tables, computer-generated sequences, or other methods that ensure allocation is not influenced by investigators or participants. Allocation Concealment: This involves hiding the allocation sequence from those involved in enrolling participants. It's vital to prevent selection bias, where researchers might consciously or unconsciously influence the assignment of participants to specific groups. Blinding: While not a part of randomization per se, blinding is closely related. It refers to keeping participants, healthcare providers, and researchers unaware of which treatment group participants are in. This prevents performance and detection biases. Common Pitfalls in Randomization Despite its importance, randomization can be implemented poorly, leading to biased results. Here are some common pitfalls: Inadequate Randomization Methods: Methods like assigning treatments based on birth dates or day of the week might seem random but can introduce systematic biases. For instance, there could be differences in care based on the day or time, making these methods unreliable. Failure to Conceal Allocation: In the past, brown envelope methods were used, where the treatment assignment was sealed in an envelope. However, this method is vulnerable to tampering. For instance, researchers might be tempted to "peek" at the assignment and selectively enroll participants, compromising the study's integrity. Small Sample Sizes: Small trials are particularly vulnerable to imbalance in baseline characteristics between groups purely by chance. This can lead to skewed results that do not accurately reflect the intervention's efficacy. Best Practices in Randomization To ensure robust and reliable results, certain best practices should be followed: Use of Reliable Randomization Methods: In modern trials, computer-generated random numbers are the gold standard. They provide true randomness and can be tailored to the specific needs of the study. Allocation Concealment Techniques: More sophisticated methods like centralized randomization, where a third party manages the allocation process, can help maintain concealment. In some studies, web-based or voice-based systems are used, which provide real-time allocation while preventing researchers from manipulating the process. Stratification and Block Randomization: To address the issue of unequal di