Building Better AI While Preserving User Privacy With TripleBlind
AI Engineering Podcast - Un podcast de Tobias Macey
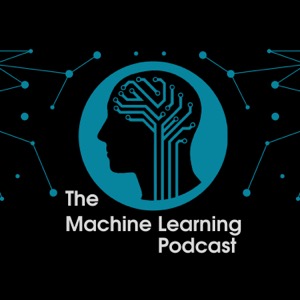
Catégories:
SummaryMachine learning and generative AI systems have produced truly impressive capabilities. Unfortunately, many of these applications are not designed with the privacy of end-users in mind. TripleBlind is a platform focused on embedding privacy preserving techniques in the machine learning process to produce more user-friendly AI products. In this episode Gharib Gharibi explains how the current generation of applications can be susceptible to leaking user data and how to counteract those trends.AnnouncementsHello and welcome to the Machine Learning Podcast, the podcast about machine learning and how to bring it from idea to delivery.Your host is Tobias Macey and today I'm interviewing Gharib Gharibi about the challenges of bias and data privacy in generative AI modelsInterviewIntroductionHow did you get involved in machine learning?Generative AI has been gaining a lot of attention and speculation about its impact. What are some of the risks that these capabilities pose? What are the main contributing factors to their existing shortcomings?What are some of the subtle ways that bias in the source data can manifest?In addition to inaccurate results, there is also a question of how user interactions might be re-purposed and potential impacts on data and personal privacy. What are the main sources of risk?With the massive attention that generative AI has created and the perspectives that are being shaped by it, how do you see that impacting the general perception of other implementations of AI/ML? How can ML practitioners improve and convey the trustworthiness of their models to end users?What are the risks for the industry if generative models fall out of favor with the public?How does your work at Tripleblind help to encourage a conscientious approach to AI?What are the most interesting, innovative, or unexpected ways that you have seen data privacy addressed in AI applications?What are the most interesting, unexpected, or challenging lessons that you have learned while working on privacy in AI?When is TripleBlind the wrong choice?What do you have planned for the future of TripleBlind?Contact InfoLinkedInParting QuestionFrom your perspective, what is the biggest barrier to adoption of machine learning today?Closing AnnouncementsThank you for listening! Don't forget to check out our other shows. The Data Engineering Podcast covers the latest on modern data management. Podcast.__init__ covers the Python language, its community, and the innovative ways it is being used.Visit the site to subscribe to the show, sign up for the mailing list, and read the show notes.If you've learned something or tried out a project from the show then tell us about it! Email [email protected]) with your story.To help other people find the show please leave a review on iTunes and tell your friends and co-workers.LinksTripleBlindImageNet Geoffrey Hinton PaperBERT language modelGenerative AIGPT == Generative Pre-trained TransformerHIPAA Safe Harbor RulesFederated LearningDifferential PrivacyHomomorphic EncryptionThe intro and outro music is from Hitman's Lovesong feat. Paola Graziano by The Freak Fandango Orchestra/CC BY-SA 3.0