AI Glossary Series: Retrieval Augmented Generation (RAG) [AI Today Podcast]
AI Today Podcast: Artificial Intelligence Insights, Experts, and Opinion - Un podcast de AI & Data Today - Les mercredis
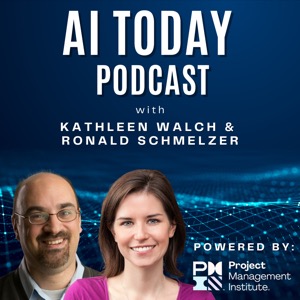
Catégories:
It’s hard to have a conversation about AI these days without the topics of Generative AI and Large Language Models (LLMs) coming up. And, Large Language Models (LLMs) have been proving useful for a variety of things such as helping write text, write code, generate images, and help augment human workers. However, as people are using LLMs, they are demanding even greater accuracy and relevance to their specific industry and/or topic area. What is a retrieval augmented generation? General purpose Large Language Models (LLMs) are trained on Petabytes of internet data and are really good at general purpose NLP tasks. These general tasks include: Text generation, Text classification and analysis, and Question answering.However, these general purpose LLMs suffer from two key problems: 1- They can only perform NLP tasks based on the data they were trained on, which might be old, irrelevant, or both.2 - These general LLMs don’t have knowledge of domain-specific, proprietary, or private data sets.How can we use the power of LLMs with its ability to understand and generate content but use our own datasets to focus content relevancy and constrain responses? This is where Retrieval Augmented Generation, also referred to as RAG, comes in. How does RAG actually work? Retrieval-Augmented Generation “grounds” the LLM system with specific, provided data sources.There are 3 steps in RAG: Retrieving data (usually text-based documents) from a source Augmenting a prompt to an LLM system using the context from the source Getting the output from the LLM system that used the augmented prompt with the added context How accurate is retrieval augmented generation? So, this begs the question: just how accurate is retrieval augmented generation? RAG has been shown to excel at providing factually accurate, contextually relevant, and brand specific responses. This should come as no surprise since it's trained on updated and relevant data. RAG is particularly useful for tasks like question answering, where reliable information retrieval is crucial. In the podcast we go over both the pros and cons of RAG. Does rag reduce hallucinations? If you've ever used an LLM you know that inaccurate responses, also called hallucinations, come with the territory. However, RAG is shown to greatly reduce hallucinations. We go into this in greater detail in the podcast and explain why. Show Notes: CPMAI Training and Certification FREE Intro to CPMAI mini course AI Today Podcast: Generative AI Series: Generative AI & Large Language Models (LLMs) – How Do They Work? AI Glossary AI Today Podcast: AI Glossary Series – OpenAI, GPT, DALL-E, Stable Diffusion AI Today Podcast: AI Glossary Series – Tokenization and Vectorization